The landscape of artificial intelligence (AI) and machine learning (ML) is transforming rapidly. Online model training and model drift management become essential for businesses to maintain competitive edges. Data streaming with Apache Kafka and Apache Flink plays crucial roles in this evolution, enabling real-time updates and seamless integration into modern data infrastructures. This blog explores the challenges of model drift, investigates TikTok’s groundbreaking architecture, and highlights the business value and complementary nature of data streaming with other platforms.
Join the data streaming community and stay informed about new blog posts by subscribing to my newsletter and follow me on LinkedIn or X (former Twitter) to stay in touch. And make sure to download my free book about data streaming use cases.
Understanding Model Drift: The Achilles’ Heel of Static Models
Real-time model inference with a data streaming platform using Apache Kafka and Flink is a powerful solution for delivering fast and accurate predictions, as detailed in my model inference blog post, but it’s not enough to sustain long-term model accuracy.
Machine learning models degrade in accuracy over time due to shifts in data or concepts—a phenomenon known as model drift.
This can take several forms:
- Concept Drift: Changing relationships between input and output variables, such as shifting user behavior.
- Data Drift: Variations in data distribution, e.g., demographic shifts.
- Upstream Data Changes: Pipeline modifications, e.g., new logging formats or unavailable sources.
Unchecked, model drift leads to poor predictions and missed opportunities. Addressing it requires continuous updates, which online machine learning enables through data streaming platforms like Kafka and Flink.
TikTok: Revolutionizing Real-Time Personalization with Kafka and Flink
TikTok’s recommendation system, detailed in ByteDance’s whitepaper, leverages a cutting-edge, real-time machine learning architecture powered by data streaming technologies like Kafka and Flink to deliver personalized content at scale, seamlessly integrating user behavior data, dynamic feature processing, and online model updates for unparalleled user engagement and platform efficiency.
What is ByteDance and TikTok?
ByteDance, TikTok’s parent company, is a Chinese technology giant renowned for its innovative use of AI and real-time ML. TikTok, its most famous product, has redefined user engagement through hyper-personalized video recommendations. TikTok employs real-time online machine learning, ensuring recommendations are dynamic, accurate, and engaging.
Why TikTok Outshines Competitors
While other social video platforms also leverage advanced machine learning for recommendations, TikTok’s architecture distinguishes itself by prioritizing real-time adaptability and hyper-personalization, ensuring it can respond to user behavior faster and more effectively than its competitors.
- User Engagement: TikTok’s recommendation engine adapts in real-time, delivering hyper-relevant content that increases user retention.
- Scalability: Unlike many platforms relying on periodic retraining, TikTok continuously updates its models, handling massive data streams with ease.
- Speed: Real-time processing reduces latency in adapting to user behavior, a stark contrast to Facebook or YouTube’s delayed batch processes.
Technical Implementation using Apache Kafka, Flink and Machine Learning for Continuous Online Model Training
TikTok’s real-time recommendation system is built on a robust streaming data architecture:
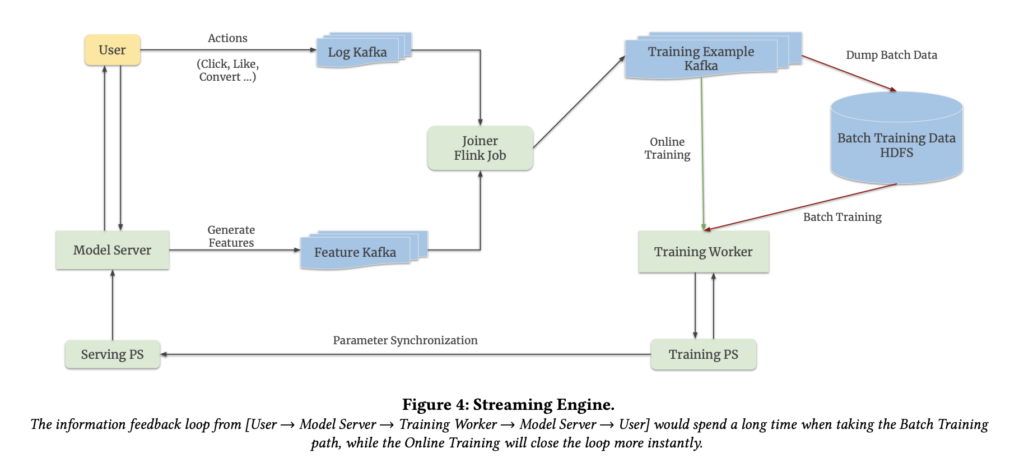
Data Ingestion:
- User interactions like views, likes, and shares are streamed in real-time via Kafka.
- Kafka ensures reliable collection and distribution of high-velocity event data.
Feature Engineering:
- Flink processes raw data streams, performing real-time feature extraction and enrichment.
- Techniques like point-in-time lookups prevent training-inference skew, ensuring the same features are used in both phases.
Online Model Training:
- Lightweight models are continuously updated with fresh data.
- This approach mitigates model drift, ensuring predictions stay relevant and accurate.
Real-Time Inference:
- Updated models are deployed immediately to serve predictions.
- TikTok’s architecture ensures latency is minimal, with recommendations delivered almost instantly.
This dynamic infrastructure has made TikTok a leader in real-time AI, setting a benchmark for others.
Data Streaming with Kafka and Flink: The Backbone of Modern Machine Learning and AI
Apache Kafka and Flink are indispensable for organizations embracing online ML.
Data streaming addresses key challenges:
- Training-Inference Data Skew: By streaming real-time features into models, Flink ensures consistency in model training and inference data.
- Multi-Model Governance: Kafka and Flink enable the data integration with small models for enrichment and large models for complex decision-making, ensuring governance and modularity.
- Scalability and Efficiency: Data streaming pipelines handle massive volumes with low latency, enabling real-time decision-making.
Complementing Other Data Platforms: Streaming Meets Analytics
Data streaming complements platforms like Databricks, Snowflake, and Microsoft Fabric, creating a seamless ecosystem for AI/ML workflows:
- Databricks: While Databricks excels in large-scale batch processing and AI model training, Kafka adds real-time data ingestion and pre-processing capabilities.
- Snowflake: Zero-ETL integration with Kafka and Flink allows for real-time analytics alongside Snowflake’s strong data warehousing and AI features.
- Microsoft Fabric: Fabric’s AI-powered analytics gain agility from Kafka’s event-driven architecture, ensuring near-instant data availability.
Shift Left Architecture with Apache Iceberg as Open Table Format for Data Sharing
The Shift Left Architecture emphasizes moving from traditional batch processing and lakehouse-centric approaches to real-time data products, empowering businesses to act on data faster and with greater agility. Learn more about this transformative approach in my Shift Left Architecture blog post.
Meanwhile, Apache Iceberg, an open table format for lakehouses and streaming, ensures seamless data sharing across real-time and batch workflows by providing a unified view of data. Dive deeper into its capabilities in my Apache Iceberg blog post.
This complementary relationship enables businesses to leverage best-in-class tools without trade-offs, providing both real-time and batch capabilities. Learn more in my comparison blog series “Data Streaming with Kafka and Flink vs. Snowflake” and “Microsoft Fabric and Apache Kafka“.
Business Value: A Compelling Case for Real-Time AI/ML with Data Streaming using Kafka and Flink
The adoption of real-time ML with Kafka and Flink drives tangible business outcomes:
- Enhanced User Engagement: Personalized recommendations lead to improved customer retention.
- Faster Time to Market: Real-time data pipelines reduce the lead time for deploying ML solutions.
- Improved ROI: Real-time adaptability ensures models deliver consistent business value.
- Freedom of Choice: Kafka acts as the backbone, enabling seamless integration with diverse tools and platforms.
This translates to a flexible, scalable, and high-performing ML infrastructure capable of handling evolving business demands.
Real-Time AI/ML with Kafka and Flink: Transforming Data into Business Value
Online machine learning with Apache Kafka and Flink is the future of adaptive, real-time AI. TikTok’s success story is a testament to the power of dynamic AI/ML systems in driving engagement and staying competitive. By complementing platforms like Snowflake, Databricks, and Microsoft Fabric, data streaming enables a holistic, future-proof data strategy.
Organizations must embrace these technologies to unlock faster time to market, unparalleled user experiences, and sustained business growth.
Let’s connect on LinkedIn and discuss how to implement these ideas in your organization. Stay informed about new developments by subscribing to my newsletter. And make sure to download my free book about data streaming use cases.